One of my goals over the next year is to analyze more of the data freely available from New York City public agencies like the NYPD, 311, TLC, and MTA.
Today, I decided to analyze the NYPD’s dataset, the Seven Major Felonies for 2015 (or technically the first nine months of 2015). I’m a big fan of the COMPSTAT releases available on the NYPD’s website, which break out crime into several categories, timeframes, locations, and shooting incidents and a comparison at the bottom of each PDF, comparing the current year against previous years (2016, 2001, 1998, 1993, 1990). Today’s dataset is going to focus on the NYPD’s ‘seven major felonies: rape, murder, burglary, robbery, grand larceny, felony assault, and grand larceny of a motor vehicle.
The data can be found here.
Interesting Findings
Felony assaults are twice as likely to occur between midnight and 4am on Saturday and Sunday.
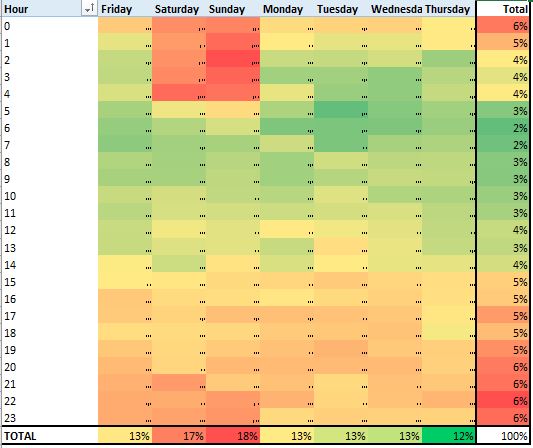
Staten Island has zero murders on Wednesday or Friday.
Bronx murders peak at 3pm, and 8pm, and 1am
Summer between midnight and 4am is when most robberies occur
8% of grand larcenies occur at noon
Murder (257)
This is category I find interesting because of the drop over the last 25 years. In 1990, NYC once average a over 6 murders per day, and ended 1990 with 2,245, this number has plummeted to (only) 352 in 2015. It’s difficult to say what caused this drop from a dataset perspective, but we are able to see trends in the 2015 data by layering on time, temperature and location.
One of my favorite quotes regarding the correlation between the murder rate and temperature came from former Queens resident 50 Cent. On his album ‘Get Rich or Die Tryin’, he postulated, “They say summer time is the killing season, it’s hot out in this bitch, that’s a good enough reason“. Fifty does seem to have a point, looking at the average number of murders that occur at a certain temperature, 75-80 degrees (Fahrenheit) is when murders peak at 45.
It’s difficult to say what causes the spike using only data, but we can make some guesses. One guess is that people may be more likely with to go outside when there’s more daylight and warmer weather. This could lead to more confrontations with friends/enemies/strangers. Another guess would be the summer vacation schedule causes more teenagers to get into trouble, an ”.
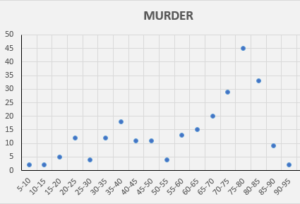
BURGLARY (10,945)
The distribution of burglary seems to be dependent on time of day. From 2pm-4am, each hour of the day will account for 5% of reported burglaries, but between 5am and 1pm, that number falls between 2-3%. I don’t have information on the location of the burglary ie: Residential vs commercial, which would be useful to know who is affected, and if the location affects the reporting. My guess is that it’s hard to determine whether these burglaries occurred at the time in the dataset, or if it’s when the NYPD received them.
RAPE (1,080)
This felony seems to be concentrated within several days and hours with several noticable spikes. 13% of rapes occur at midnight, in terms of weekday, Saturday and Sunday make up 18 and 17% respectively. There are small spikes in the data at 4am, 8am, and noon.
Grand Larceny of a Motor Vehicle (5,515)
I’ve only briefly looked at this felony, but it appears that half occur between 6pm and midnight. Breaking it out by month, 27% of GL’s were commited in August and September, while only 17% occured in January and February.