Lifetime value is an important marketing concept which describes the amount of money a business can expect earn from a customer. For this post, I’ve decided to calculate how much I’m worth to a business over the last 12 months using all my spending using Mint.com.
Business Analysis
I was really interested in the businesses where I spend the most money. For this analysis, I have three categories essential expenses costs (rent, public transport, phone, groceries), and non-essential expenses (alcohol, coffee, fast food). I’ll ignore the essential expenses because nobody really cares that I spend the same amount every month with the MTA or Verizon. Below is a handpicked list of businesses that I spent lots of money at last year.
BLACK STAR COFFEE, $1,025: The business that surprised me the most was Black Star Coffee. The average meal for me is a croissant, and a double cortado on weekdays, and a siracha egg sandwich and iced coffee on weekends. Before looking at the data, I expected my monthly spending to be around $40. Using Mint, I saw that over the last 12 months, I spent over $1,000 on 136 purchases (average cost of $7.54). Clearly I was way off, I was blindly handing my credit card over without actually doing the math in my head, of how often I frequented this coffee place.
I like their croissants, and breakfast sandwiches a lot, I highly encourage people to try it out. But I can’t justify spending at a conservative estimate, $400 a year on coffee from one place. I could buy a coffee machine for $40, and pay an average of $0.40 for a freshly brewed cup of coffee, saving myself around $3.50 per cup.
TRADER JOES WINE SHOP, $409: Over the last year, I’ve really gotten into Trader Joe’s “two buck chuck”, officially known as Charles Shaw Wine, which is available for $3 for one bottle. From the data, I make a bi-monthly trip to the Trader Joes wine shop, where I pick up two bottles the first is usually an $8 Malbec, and the second is either a bottle of two buck chuck, or a second $8 Malbec, all of which leaves me with an average basket of $16.35 (incl. 10% NYC tax).
I expected my spending to be much higher with Trader Joes Wine, I was under the impression I visited more often and spent more in an average visit. In terms of cost reduction, there’s not much I can except stop drinking. In my opinion the wine is a great value, it doesn’t taste like it’s two dollars and it’s better than most beer.
Seamless, $714: I was really worried that Seamless would be over $1,000. I estimated that my average basket was between $18 – $21 (actually $19.85), and that I ordered around 50 times (actually 36 times). I’m fairly loyal, 2 restaurants accounted for 44% of all orders. Half of all orders came in the final three months of 2016, while the other half came over the next nine months. It’s good to know that I learned self control
One interesting to note, in 2016 I spent $979 on 52 orders, I’m not proud of it, but it was so convenient.
Category Analysis:
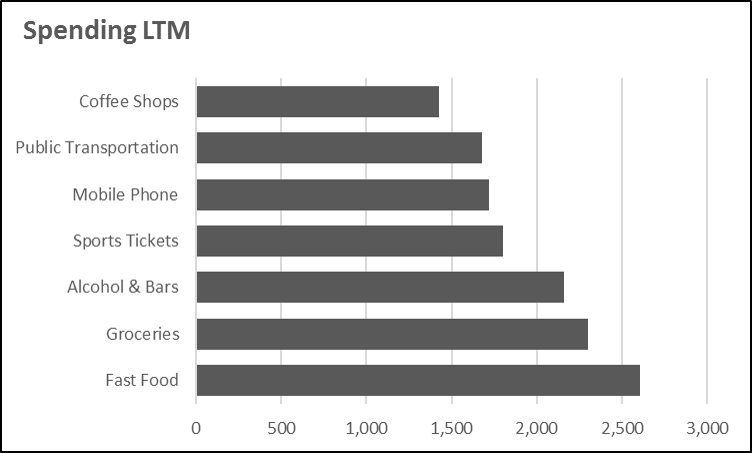
Fast Food $2,606: This is pretty embarrassing for two reasons. First these costs are mostly avoidable if I brought lunch, or stopped using Seamless. Bringing lunch twice a week could save $1,000. Second, I spent less on groceries than fast food. Every time I buy lunch, I spend an average of $10.47, but cooking at home could cost me around $2 – $3 which is an 80% decrease in costs.
Sports Tickets $1,804: I’m a huge sports fan, over the past 12 months I went to the French Open, US (Tennis) Open, NHL Rangers games, and several soccer games. Over the next 12 months I expect this to remain the same or slightly increase because I’d like to attend more NHL games, and an NFL game. It’s not cheap, I spend around $150 dollars on each event (excl. soccer).
Television and Streaming $549: I use Sling TV, HBO Now, and Netflix Streaming for all of my TV and streaming.
Does this satisfy all my viewing needs? Technically yes, the only thing I’m missing certain sports networks like YES, FS1 and MSG. The channels that I do watch live are available in Sling and HBO Now: ESPN, Comedy Central, Adult Swim, HBO, and I can watch certain sports using a $15 (one time cost) digital TV antenna.
For comparison, using cable like Spectrum I could pay around $130/month, putting my total spend to $1,560 + $203 from Netflix.
Next Steps
Some areas were eyeopening. I spend way too much on greasy Seamless food and lunch. If I do spend money, it should be on nicer restaurants that I visit with friends. Coffee was another unnecessary expense that I regret. Coffee was usually ordered with a snack or sandwich which often doubled the cost. For the next 12 months I should focus on brewing my own coffee, eating out less for dinner and bringing my lunch which could easily save me $1,000. One area that I don’t expect to change, is sports tickets and alcohol. I really enjoy seeing sports live and I don’t expect to change that anytime soon. I would like to spend less than $2,000 over the next year, but realistically with dates, happy hours and NYC’s obsession with cocktail bars it will be hard. I think it makes sense to track these expenses closer in Mint. I’ve set up budgets which I’m trying to follow a little more closely, and I’ll try and do a quarterly post-mortem of expenses to see if I followed through on the cost reductions.
Source: Mint, Excel used for Viz